The LAPD Is Spending Millions On Spy Tech to Beef Up Security
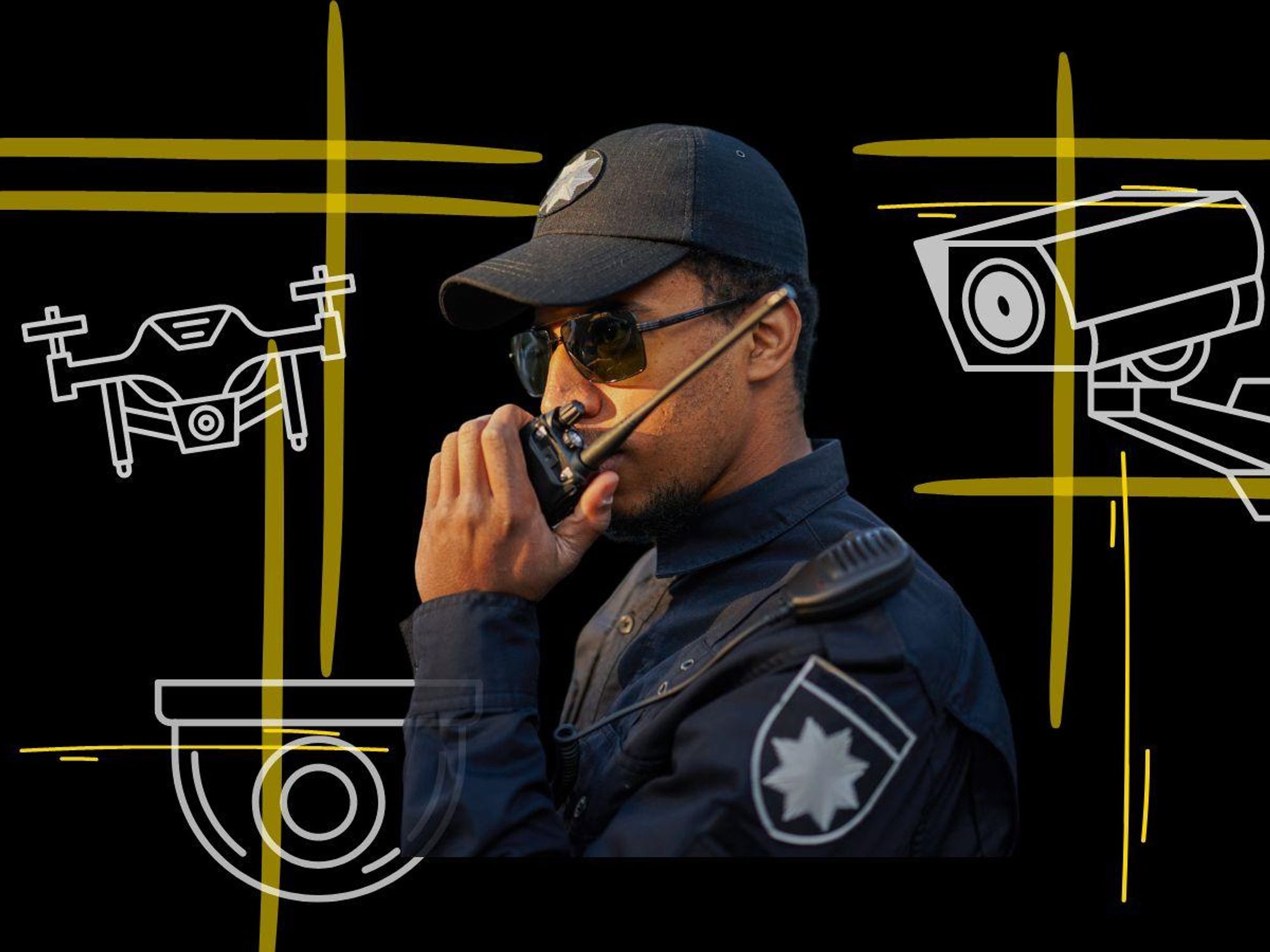
Over the past six years, the LAPD spent millions in FEMA funds on automated license plate readers, predictive policing software and other spy tech, according to a new report. Authored by Action Center on Race and Economy (ACRE), the report focused on a counter-terrorism grant program under FEMA known as the Urban Area Security Initiative (UASI). First created in 2003, the UASI was designed to help the largest cities beef up their emergency preparedness agencies and prevent acts of domestic terrorism.
According to a mayor’s report from January 2021, the city of Los Angeles received roughly $20.5 million in UASI grants. Of that amount, approximately half (or $10 million) went to the LAPD. Notably, the amount was only a drop in the bucket of LAPD’s total $1.7 billion budget for the fiscal year 2020 (which was cut by $150 million in response to the movement to defund the police). For fiscal years 2022-2023, the L.A. City Council approved a $1.9 billion operating budget for the city’s police.
While local police departments receiving federal money is nothing new, critics say the existence of such funds gives the LAPD more freedom to invest in invasive technologies. LAPD recently came under scrutiny for its use of facial recognition technology. Earlier this month, an inspector’s general report revealed that the LAPD’s use of facial recognition software only resulted in a positive match about 55% of the time and the department didn’t track incidents where matches led to a misidentification of a suspect.
Automatic License Plate Readers (ALPR)
Between 2016 and 2020, the LAPD purchased at least $1.27 million worth of ALPR readers per report. ALPRs have increasingly come under increased scrutiny for their high error rates and risks to privacy. The high-speed cameras scan images of nearby license plates and alert police officers to stolen vehicles or people wanted for a crime.
One 2020 audit found that the LAPD and three other police departments were collecting massive amounts of data on drivers and their movements — but weren’t doing enough to protect privacy. According to the audit, of the 320 million images that the LAPD had stored in 2020, roughly 99.9% were unrelated to criminal investigations.
Palantir Data Fusion Platforms
Palantir, a controversial software company that has faced criticism for enabling government surveillance, has provided predictive policing software to the LAPD since 2011. The company’s platform can identify criminal “hotspots” by analyzing license plate photos, police reports, gang databases, regional crime reports and other data.
The exact amount of money the company has received from UASI is unclear. Public records requests, however, estimate that the LAPD spent over $20 million on Palantir software between 2009 and 2018.
It’s important to not that such tools have disproportionately targeted low-income individuals, people of color and unhoused people. Last year more than 1,400 mathematicians signed on to a letter criticizing predictive policing for its racial biases in the trade journal Notices of the American Mathematical Society (AMS). In 2019, PredPol (a predictive policing tool once used by the LAPD) faced criticism from mathematicians for using flawed algorithms that created feedback loops.
“If you build predictive policing, you are essentially sending police to certain neighborhoods based on what what they told you—but that also means you’re not sending police to other neighborhoods because the system didn’t tell you to go there,” University of Utah computing professor Suresh Venkatasubramanian toldMotherboard.
Radio Systems
Between 2016 and 2020, the LAPD spent roughly $24 million to upgrade their radio communications network through Motorola. As the Los Angeles Times reported in 2007, the department’s two-way portable radio system was often unreliable and had been in need of an upgrade for years. Some officers even resorted to using their cell phones for field communications.
Critics noted that radio systems have allowed police to avoid public oversight in light of many cities encrypting their scanners in recent years. Furthermore, since 2020, many cities including Santa Monica, Santa Cruz, San Diego and others (though not Los Angeles) have opted to take their radio communications private in order to comply with a DOJ directive to protect private information. But critics warn that such a move prevents the media and the public from keeping track of criminal activity or public safety developments during natural disasters.
Cell Site Simulators
The LAPD also spent $630,000 of 2020 UASI funding on cell-site simulators — devices that look like cell towers that allow police to pinpoint the location of a specific smartphone. Cell site simulators can identify the unique IMSI number (international mobile subscriber identity) attached to every mobile device.
Also known as Stingrays or IMSI catchers, the devices can collect the content of SMS messages, voice calls and any websites visited. IMSI catchers essentially trick nearby mobile devices into connecting with them and then collect the data sent from the device, including its location and other personal data. Some devices even have the ability to intercept text messages and phone calls.
Cell site simulators are in ample use in California and and in major police departments throughout the country, including in cities like Chicago, Boston and New York City. Critics of cell site simulators say they function as dragnet surveillance tools — essentially capturing data from bystanders—and also can potentially interfere with 911 calls.
Social Media Surveillance
Skopenow — a social media monitoring company — counts the LAPD as one of its customers, along with the U.S. Secret Service, the U.S. Postal Inspection Service and Broward County, Florida. Last year, the software led to the arrests of three middle school students in Florida after police found threats they made on social media.
According to the company’s website, Skopenow functions as a sort of “analytical search engine” for social media. It claims it can inform customers when criminals post content related to drugs, weapons or stolen items. It also lets users easily view a person of interest’s mutual friends, shared vehicles, employment histories and business affiliations.
Since the ACRE report’s analysis doesn’t go past fiscal year 2020, it doesn’t capture recent developments in LAPD’s use of surveillance. In August, the L.A. Police Commission adopted rules that will require the LAPD to submit detailed proposals before acquiring new technology. It also needs to disclose which data will be collected on people and for how long it will be kept.
While such reform is definitely a start, critics point out that similar policies on facial recognition haven’t reigned in police abuse and have instead served as a cover.
- After Dozens of Wrongful Arrests, a New Bill Is Cracking Down on Facial Recognition Tech for Law Enforcement ›
- In LA, the Fight Over Facial Recognition Tech Is Just Heating Up ›
- Inside the First-Of-Its-Kind TikTok Task Force To Stop Kia Theft ›
- LAPD Faces Criticism After Using SweepWizard - dot.LA ›